C-Store Leaders: AI is Accelerating Convenience Retail Analytics
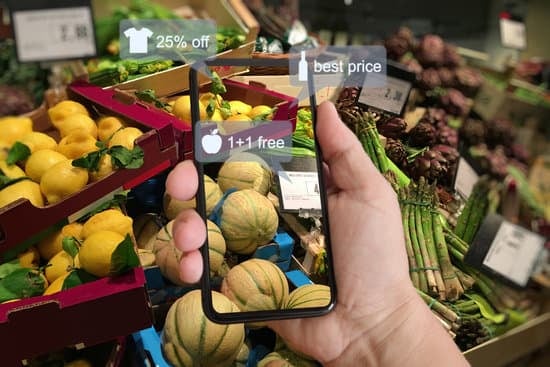
C-store industry leaders issued a new report that says generative artificial intelligence (AI) has unleashed a new era in retail analytics.
“Evolution of AI sharpens the focus of convenience channel operations,” reported Convenience Store News. “Retailers are exploring its use in inventory optimization, fuel pricing, and more.”
The Convenience Leaders Vision Group (CLVG) – convenience retail pioneers and trendsetters that hold quarterly virtual meetings – issued a June 2023 “Vision Report” that takes a deep dive into generative AI (including the new large language models such as ChatGPT) and its impact on convenience channel operations.
“Understanding the full potential of this new, elevated AI is no small task, but many contend that all executives — tech titles or not — should initiate their deep dive into the potential of generative AI,” said the Vision Report. “Many believe that when we look back in history, we’ll think of this time period in 2023 as the dawning of a new era in analytics.”
Defining Terms: AI vs. Generative AI
It helps to understand the key terms such as:
- Artificial intelligence (AI): intelligence exhibited by machines, with machines mimicking cognitive functions that humans associate with other human minds.
- Machine Learning (ML): the major approach to realize artificial intelligence by learning from and making data-driven predictions on data and experiences.
- Deep Learning (DL): a branch of Machine Learning where a set of algorithms attempt to model high-level abstractions in data.
“From a top-line point of view, distinguishing between generative artificial intelligence and broader artificial intelligence is far less important for non-tech executives than simply having a true understanding of what is possible. In simple terms, generative AI is a form of AI,” says the Vision Report.
A basic difference between AI and generative AI:
- AI systems process human-like intelligence.
- Generative AI models leverage that with deep learning to create new content in the form of images and text.
McKinsey & Co. analytics partner Tom Svrcek, who presented to the CLVG as part of the Vision Report, likened generative AI to the way one learns to drive a car in the rain.
Svrcek: “When learning to drive in the rain, it’s common to hit the brakes too hard, which causes the car to hydroplane, which in turn teaches the driver not to hit the brakes too hard, and that person will likely make a different decision the next time they are driving in the rain. Essentially, it is about receiving information, acting on that information, trying something, subsequently succeeding, or failing, learning from that, and applying that learning the next time. That constant series of pattern recognition is how AI works.
So, like how the human brain works, an AI model works in a circular fashion like this:
- Receive Information: Machines do tasks or make decisions based on the output of algorithms and their predictions.
- Learn: Machines process the data through algorithms, changing them as they learn more about the information that they are processing.
- Decide: Machines do tasks or make decisions based on the output of algorithms and their predictions.
“Learning from either the success or failure of those actions enables AI to do what it does,” Svrcek added.
How AI is Already Disrupting Businesses and Society
The McKinsey report delivered as part of the Vision Report highlighted how AI is already disrupting businesses and society.
Consider these facts:
- 425 percent increase in VC investment in generative AI since 2020.
- $70 billion in global AI funding in 2021.
- 370+ AI U.S. startups, exceeding $10 billion in total capital raised.
- $300+ billion expected enterprise revenue from AI in 2025.
- 1 million users in 5 days for ChatGPT (generative AI).
Why now? McKinsey says three trends have converged to put AI in a position to have an immediate and practical impact on all businesses:
- Tumbling costs of data storage and processing.
- Rapidly expanding data availability.
- Advance new algorithms from AI to ML to DL.
AI’s Transformative Impact Across C-Stores Operations
The CLVG Vision Report slide deck provided by analytics partner McKinsey & Company said that AI is having a transformative impact across traditional C-store levers – from commercial to operations – as well as creating new-to-world value.
Some of the ways that AI is impacting convenience store analytics include:
- Inventory Optimization: Predictive machine learning models are dramatically improving inventory management across the entire supply chain.
- Assortment and Space Planning: Hyper-localization of product assortment and space planning driven by new and unconventional data sources, are maximizing the value for stores.
- Pricing and Promotion: AI-driven dynamic pricing and promotion models -- both within the store and at the pump – are enabling previously infeasible levels of personalization.
- Innovations in Security and Customer Experience: Computer vision-assisted video analytics are significantly increasing store security and providing valuable new behavioral insights on shoppers.
Case Studies: Improving Inventory Optimization and AI-Driven Dynamic Fuel Pricing
The Visions Report shared use cases where predictive learning algorithms are improving inventory optimization across the supply chain, and AI-driven dynamic pricing, promotions optimization, and checkout drive strong sales and margin growth.
How AI improves inventory optimization across the supply chain:
- Products on the Shelf: On-shelf availability – collect video and track stock, detect damaged or mislabeled items, and analyze and forecast peak and non-peak demand. Machine vision can detect anomalous demand in near-real time, enabling alerts to DCs or supplies, increasing availability. This increases sales via better visibility and precisely manages total inventory and reduced shrinkage.
- Store Inventory Management: Demand forecasting – detect early signs of increased demand and inform replenishment decisions to the store manager. ML-based forecasting algorithms can test tens of millions of demand signal hypotheses to dramatically increase accuracy. This prevents sales loss from stockouts and reduces inventory costs from overstock.
- Product Replenishment: Automated store replenishment – deploy short-term, and mid-term demand forecasting, optimize stock for high availability, balance inventory and purchase cost via order quantities. Dynamic, automated decision-making solutions can analyze demand and supply, consider order quantities, and auto-refill inventory. This reduces total inventory costs.
- In-Warehouse Fulfillment: Inventory tracking – improve product tracking, and inventory audit process via high-definition computer vision. Computer vision can scan and read barcodes to track all inventory and movement. This improves tracking accuracy and reduces labor and manual tracking processes.
AI-driven pricing, from the pump to checkout, has the potential to:
- Fuel Price Dynamic Pricing: Obtain competitors’ pricing, segment customers, predict elasticity and demand, and adjust site-by-site pricing multiple times in a day. Test and learn to improve algorithms over time. AL-based algorithms learn granular price sensitivities and willingness-to-pay to drive solutions that maximize revenue. This increases sales via optimizing price and volume and increases customer traffic for retail.
- Personalization At-Scale: Push customized promotions and offers to the right customers at the right time. Retain existing customers and acquire new customers through digital marketing. ML-driven algorithms can identify customers' purchase patterns and “lookalike” customers can be found through collaborative filtering techniques. This increases sales through targeted promotions and increased traffic. It also prevents customer churn and acquires new customers.
- Retail Price and Promotion: Revenue growth management by segmenting customers based on past purchases and demographics. Predict customer elasticity and determine optimal pricing and promotions (next best products, frequency, depth, etc.). Micro-segmentation can predict price sensitivities and the likelihood of jointly purchased SKUs, while reinforcement learning can help with optimal RGM strategies. This improves sales via optimizing price, volume, and upsell/cross-sell.
- AI Automatic at Checkout: Self-serve technology enables reduction/replacement of frontline personnel away from repetitive tasks toward driving customer experience (e.g., “the concierge”). Machine vision can be employed to prevent an increased likelihood of shoplifting. Automation and interpretive video analytics can combine to improve service times while also managing risks. This improved productivity while increasing resources focused on CX.
“The ability to set gasoline pump prices based on individual customer behavior offers a whole new opportunity,” noted Greg Parker, CEO, Parker’s Kitchen.
Kevin Smartt, CEO of TXB Stores, agreed with Parker on fuel pricing and AI.
“I’ve looked at some AI-based programs for fuel pricing, which is really looking at pricing in the future instead of pricing from behind. So it’s knowing based on the past, if you make a movement, what’s really going to happen in the future and say, most companies price their fuel based on what’s happening on the street today, but the AI’s telling you based on what it thinks is going to happen at the end of the day and tomorrow, so you’re moving your price ahead of time, which is really an interesting thought process when you think about it. So I think that probably has some pretty big application to our industry as the cost comes down and it gets worked out,” said Smartt.
When asked if this AI technology is an application for C-stores right now or two years down the road Svrcek said that many applications are feasible now on an economic scale.
“My personal opinion is that there are a lot of things that are very much today. The fuel pricing example is a great one. As long as you have a technology that allows you to physically change the price or charge somebody differently, either an offer or change the price that is displayed, you can do this today,” said Svrcek.